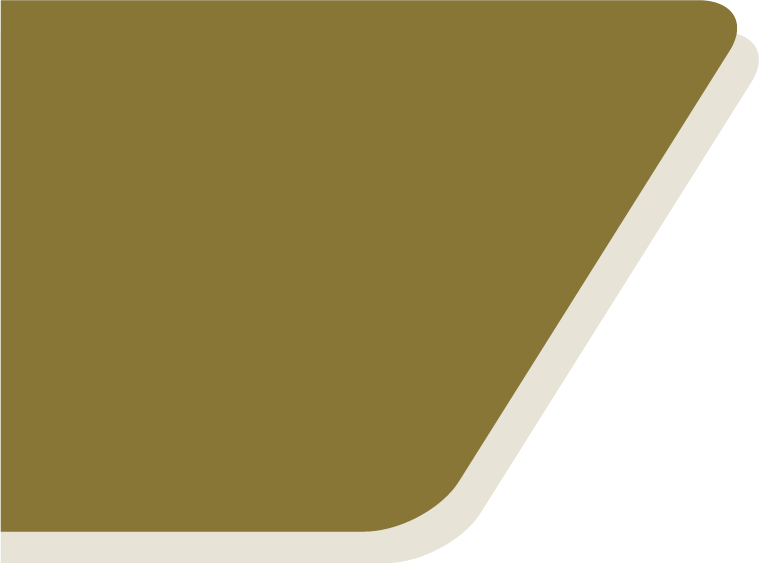
Multivariate Analysis
This module provides an introduction to multivariate analysis, with a strong emphasis on data visualization by means of multivariate graphics known as biplots. The course covers principal component analysis (PCA), multidimensional scaling (MDS), correspondence analysis (CA), canonical analysis, cluster analysis, discriminant analysis (DA) and some multivariate inference, illustrating these methods with genetic data.
Some genetic datasets have a compositional nature, and basic principles of compositional data analysis like log-ratio transformations are considered. The use of multivariate methods for uncovering population substructure and cryptic relatedness is also addressed.
Learning Objectives: After attending this module, participants will be able to:
- Describe the purpose of basic multivariate statistical methods.
- Select an appropriate multivariate method for a given data set.
- Apply adequate transformations for a given data set.
- Perform multivariate statistical analysis on a computer in the R environment.
- Visualize multivariate data by means of biplot construction, and carry out basic multivariate hypothesis tests.
- Interpret biplots correctly and assess goodness-of-fit.
- State the peculiar nature of compositional data, and account it for in the analysis.
Course Dates
- Mon June 10, 8:30 a.m. – 5:00 p.m. EST
- Tue June 11, 8:30 a.m. – 5:00 p.m. EST
- Wed June 12, 8:30 a.m. – 12:00 p.m. EST
Instructor
- Jan Graffelman
- Learn more about the instructor.
Suggested Course Pairings
Statistical Analysis Stream
- Module 1: Probablity and Statistics
- Module 5: Bayesian Statistics
- Module 9: Statistical Genetics
- Module 19: Association Mapping
Course Materials
Visit the Box here
About the Instructor
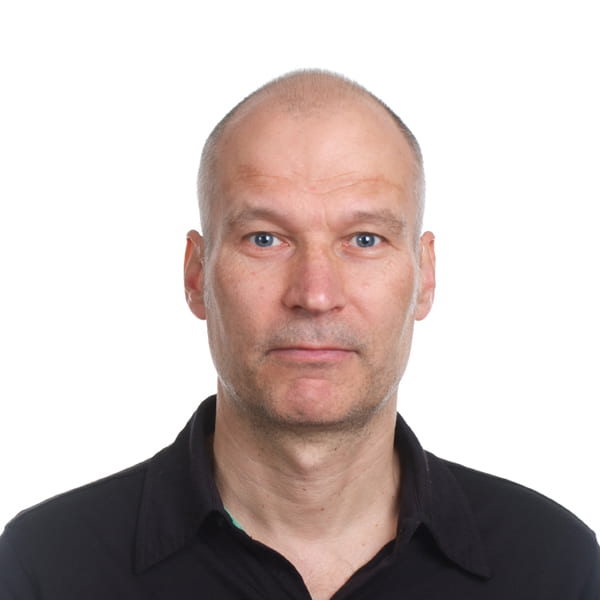
Jan Graffelman is a Associate Professor of Statistics and Operations Research at the Universitat Politècnica de Catalunya in Barcelona. His area of research and software development includes biostatistics, statistical genetics, multivariate analysis, and compositional data analysis. Learn more about Jan’s work here.